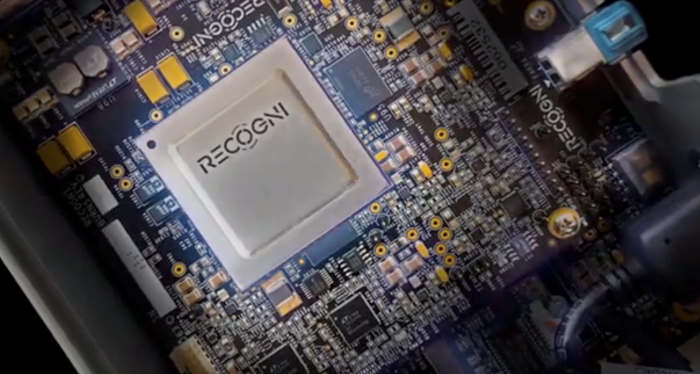
Achieving the performance standards necessary for SAE Levels 3 – 5 driver autonomy at lower costs requires a fresh approach. Can you explain a little about Recogni and its route?
Any advances towards SAE Levels 3-5 at mass consumer vehicle scale will require the autonomous systems to have incredibly high levels of computational capacity while consuming minimal energy – all at incredibly low cost. Solving all three demands simultaneously is a difficult task.
The primary mode of perception for autonomous vehicles is visually using cameras. The use of cameras is a prerequisite for autonomous vehicles – because it is the only mode of sensing where AI-based processing can recognize and classify objects i.e., pedestrians, cars, traffic lights, a traffic sign that means something like 40 mph.
To this point, cars have been designed with cameras that have resolutions in the 1-1.25 MP range (MP – megapixel). This is not because as an industry they have been constrained to use low-resolution cameras when we have 8-12 MP cameras in our phones. The primary issue is that there is no corresponding computational platform that can process these 8+ MP streams efficiently and at low power.
Recogni’s focus has been to architect a system (ASIC + software) that has the world’s most power-efficient capability, but also simultaneously brings an order of magnitude jump in performance so that multiple high-resolution and high frame-rate camera outputs can be processed simultaneously.
Once camera-based AI Inference systems with the performance metrics that Recogni provides, in conjunction with inexpensive RADARS, a total solution, we are well onto the path to ubiquitous higher levels of autonomy.
How well do you really know your competitors?
Access the most comprehensive Company Profiles on the market, powered by GlobalData. Save hours of research. Gain competitive edge.
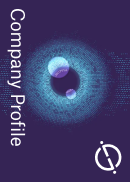
Thank you!
Your download email will arrive shortly
Not ready to buy yet? Download a free sample
We are confident about the unique quality of our Company Profiles. However, we want you to make the most beneficial decision for your business, so we offer a free sample that you can download by submitting the below form
By GlobalDataWe understand that Recogni has raised funds recently. How will the money be invested?
Recogni raised $25M in June 2019 as part of a Series A round – with GreatPoint Ventures, Toyota Ventures, BMW i Ventures among a group, being part of that investment cycle. In January 2021 Recogni raised $48.9M in a Series B round – with Celesta Capital, Mayfield, Bosch and Continental as investors in addition to Series A investors joining in.
What are the challenges of AI-based vision processors in their current form? And how do you see this marketplace evolving?
The current set of AI-based vision processors has enabled the industry to get to this point. Autonomous vehicles massively benefit from higher and higher resolution cameras – as they only make the perception more robust and dependable. Incredibly good perception is the prerequisite foundation for the superstructure of the autonomous software and system that is built over it.
As camera sensor resolutions go up – the compute demands to process this data will increase non-linearly. Being able to keep up with this will be very important.
Improving AI vision-based compute capacity is a function of the chip/system architecture – and if one is incrementally building upon legacy or general-purpose prior designs – the improvements will only be incremental. A fundamental rethink is needed in the architecture and implementation of this class of computing and once you have a much more capable starting point – then building upon that with technology and process geometry improvements becomes that much easier.
The software-defined architecture is opening up new business models for automakers. What’s your vision of the opportunities?
By 2025-26 a significant portion of a vehicle cost will be electronics. To be able to extract the maximal performance of these digital processors and systems – the vehicle has to be architected with a software-defined approach – which enables significant modularity and allows for asynchronous replacement of older components with newer & beefier systems without compromising functionality or safety.
Once the vehicle has all the capabilities to be fully software-defined – it will now enable the auto manufacturers to deliver new applications that are monetizable, and most importantly – enable features on demand – so that consumers can pay for per-use kind of features – even for autonomous capabilities. Imagine a vehicle that has inherent full autonomous capabilities – but the consumer chooses to enable it for a long highway drive whenever they want to.
We are hearing that while manufacturers remain excited about automated driving, the challenge will require more time and effort to fully realize. What’s your view on the path towards AD?
Credit has to be given to the whole industry and ecosystem to have made progress to this point. Getting to fully autonomous systems will still take more time – but certain key technology breakthroughs are happening constantly that accelerate that journey.
As an example – the level of maximum compute and compute/watt that Recogni is building can become one of those key “best of breed” technologies that speed up the momentum towards full autonomy.
Do you expect to see a bigger role for simulation as part of the training, validation and testing of AVs?
The number of real-world scenarios and settings are infinite when it comes to autonomous vehicles. One cannot plan or expect all these scenarios to be played out in the real world – while driving/testing autonomously – and then used to improve the technology.
Simulation, exceptional synthetic environments, scenario creation tools, manipulating road and weather conditions – are all tools that will enable more robust training, validation and testing of AVs – even before a single mile is driven on a real road. These tools are getting more and more sophisticated every day and make the task of modelling easier, randomizable and varied.
I guess developing solutions for autonomous vehicles is just the first step for Recogni. What other sector opportunities are you looking at?
For real-time systems to navigate and traverse the world autonomously, visual comprehension of their environment is paramount. Recogni’s technology is designed for the most demanding of these days – autonomous vehicles in the most complex roads and traffic situations, real-time in nature and incredibly responsive.
These capabilities are nicely applicable to equally demanding scenarios where visual understanding is needed – be it in robotics, high-speed material handling, factory automation, drones and UAVs and many other situations. At this point – Recogni is keenly focused only on the automotive space.