
Autonomous vehicles (AVs) rely on artificial intelligence (AI) to direct behaviour. AI is built up through machine learning in which algorithms are fed with millions of data points yet this process is currently creating a bottleneck for the industry. Continuing just-auto/AIC's series of interviews, we spoke to Matt Daley, managing director of rFpro, about how his company is addressing it.
What is the biggest challenge in training autonomous vehicles?
Currently, many players in the autonomous vehicle field employ an army of people to manually annotate each frame of a video, LiDAR point or radar return to identify objects in the scene to create training data. This covers everything from vehicles, pedestrians, road markings to traffic signals. It usually takes around 30 minutes per frame and incurs a 10% error rate, so generating reliable training data from real-world recorded scenarios is incredibly expensive, time inefficient and inflexible. The industry has a desperate need to create high quality, simulated training data which can complement existing real world recorded data, to speed up the machine learning process.
What can be done to address this?
Delivering dependable simulated training data requires several key components, such as the vehicle model, sensor models, traffic simulator and the digital world content to work together, not to mention the necessity of a robust simulation process to harness the available computer power. At rFpro we have developed a new approach that slashes the hardware costs associated with large-scale simulation. This enables customers to build complete datasets that cover the full vehicle system with every sensor simulated at the same time. The data is synchronised across all sensors, even with the most complex hardware designs. We call it Data Farming because it's comparable to Render Farming, which has revolutionised the economics of popular animation.
What does Data Farming achieve?
How well do you really know your competitors?
Access the most comprehensive Company Profiles on the market, powered by GlobalData. Save hours of research. Gain competitive edge.
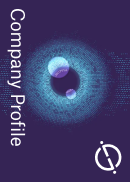
Thank you!
Your download email will arrive shortly
Not ready to buy yet? Download a free sample
We are confident about the unique quality of our Company Profiles. However, we want you to make the most beneficial decision for your business, so we offer a free sample that you can download by submitting the below form
By GlobalDataCompared to manual annotation, it provides a digital, cost-effective way of creating the same data completely error-free and 10,000 times quicker. Such a step-change in output enables deep learning to fulfil its true potential because it significantly reduces the cost and time of generating useful training data.
The simulation can be run either faster or slower than real time where there is no requirement for Driver or Hardware in the Loop (DIL or HIL) operation. Running slower than real time permits fewer processors, of lower specification, to produce the same high-quality data. Alternatively, simple data, such as that from a single sensor, could be run much faster than real time to produce results more quickly.
Does it require additional investment in computing hardware?
Decoupling the processing activities from real time operation actually removes the need for large numbers of high-end processors and enables the user to reset the balance between cost, speed and data quality to suit their particular priorities. For example, customers can start with just a single PC yet perform a complex simulation involving multiple sensors. For engineers, this puts it within a typical departmental budget, rather than requiring senior approval, making high quality training and test data far more accessible.
Is the industry ready to accept the change?
Data Farming is already being utilised by some of our existing customers. Global Tier1 supplier, Denso ADAS Engineering Services has used the process to create an extensive number of driving scenarios. And with it being a virtual platform, each scenario has an almost limitless amount of variations that can be applied. Denso found it could quickly and cost-effectively generate the high-quality training data essential for developing neural networks for its autonomous vehicle technologies.
Another customer that has successfully employed Data Farming is Ambarella, a leading autonomous vehicle technology provider, for whom Data Farming is already speeding up the development of autonomous vehicle systems. Ambarella have told us that in fact it may not be realistically possible to get to the standard required using manually annotated data sets alone. I think that indicates how vital the AV industry requires a digital alternative.
To what extent can your approach accelerate the development of AVs?
This is really dependent on the particular customer and their appetite and requirement for training data. As simulations are parallelizable, the quantity of training data that can be produced is only limited by the computing power available. In simple terms, the more computers you have the more simulations can take place.
We estimate that our approach is up to 10,000 times faster than traditional manual annotation commonly used within the industry. Perhaps more importantly, because we control the digital environment that the scenarios are created within, it is completely error free.
We understand that AB Dynamics acquired rFpro last year. In what ways have they supported the development of this new approach?
AB Dynamics has been very supportive of our new approach, of course. As well as the owner they are also a user of the software and we work closely with all our customers to ensure we are developing relevant technologies for the industry.
We are hearing that while manufacturers remain excited about automated driving, the challenge will require more time and effort to fully realize. What's your view on the path towards AD?
It has become very clear that the challenge of automated driving is even bigger than the industry first expected.
It has become very clear that the challenge of automated driving is even bigger than the industry first expected. It is also increasingly clear that real world testing alone cannot subject autonomous systems to the variety of scenarios that is required for development. It is also too expensive and time consuming to rely on collecting data and testing in the real world. The answer, as we see it, is more and more high-quality simulation that is then correlated using real world testing.
Is there a common industry approach to defining scenarios that AVs will face?
There are many players in the industry working on ways to create a common scenario description language. We believe this is important so that the industry as a whole, and all of its differing simulation methods, can execute the test in the same way, using this language. As well as simulation companies such as rFpro, those involved in this range from governments, standardisation bodies, road safety agencies and vehicle manufacturers. Our efforts to achieve this have also been bolstered by AB Dynamics Group joining the Association for Standardization of Automation and Measuring Systems (ASAM), a non-profit organisation that promotes standardisation for tool chains in automotive development and testing.
What is your vision of how the industry is likely to develop testing regimes for AVs? i.e. do you see future legislation and vehicle safety testing requiring ADAS systems and AVs to be validated in increasingly complex scenarios?
Yes, and in fact this is already happening. The amount of ADAS test scenarios in the Euro NCAP assessment has increased by 650% since 2014. We expect this trend to continue and the breadth and complexity of these types of industry tests to grow rapidly. As a result, the testing industry is already moving towards a large proportion of these tests being simulation based, with a sub-set being correlated back to real-world "control tests" to give confidence in the simulation results.