
With driving simulators becoming increasingly realistic, allowing more tests to be effectively undertaken in a virtual environment, growing numbers of proving grounds are investing in ‘digital twins’ of themselves. To learn more about what is accelerating this trend, we spoke to asked Chris Hoyle, from simulation software company rFpro.
Real world autonomous vehicle development has been somewhat halted since a fatal Uber accident last year. This has put greater emphasis on using the virtual world to train the AI systems required for these incredibly complex vehicles. In order to correlate the data and show the virtual world accurate represents the real world, proving grounds have been investing in digital twins of themselves. The Digital Twin provides a safe, repeatable way of carrying out increasingly complex scenarios in a controlled environment. rFpro believes that this will form the basis of autonomous vehicle approval for real-world tests. Hundreds of thousands of scenarios from a comprehensive library will be tested in the virtual world, followed by a random sample of these tests accurately represented at a proving ground to prove correlation.
Why would you want a digital model of a Proving Ground?
Using a Digital Twin of a proving ground helps vehicle manufacturers to accelerate development, particularly of ADAS and CAVs (Connected Autonomous Vehicles) by testing them in a fully representative virtual environment before validation on the actual track. Testing the systems in the laboratory shortens the ADAS test cycle because new control systems, algorithms and sensor models can be tested and calibrated in simulation. The savings in time and cost are significant over the life of a project because virtual testing is so much more productive than physical testing.
Testing in a virtual environment is the only cost-effective way to introduce self-learning systems to the limitless number of scenarios that can occur in the real world.
Testing in a virtual environment is the only cost-effective way to introduce self-learning systems to the limitless number of scenarios that can occur in the real world. Most AI systems, in particular those that deploy supervised learning, learn by experience. Their training data sets must continually grow to include any new types of situation that might be faced in the real world. Training using real-road data is too slow and testing on the public road is too risky. To ensure that the simulation is truly representative, correlation must take place in the real world. Proving grounds that invest in a Digital Twin become a critical part of the vehicle development process, future proofing the business.
But isn’t the emphasis among CAV developers to be ‘first on the road’ with their technology?
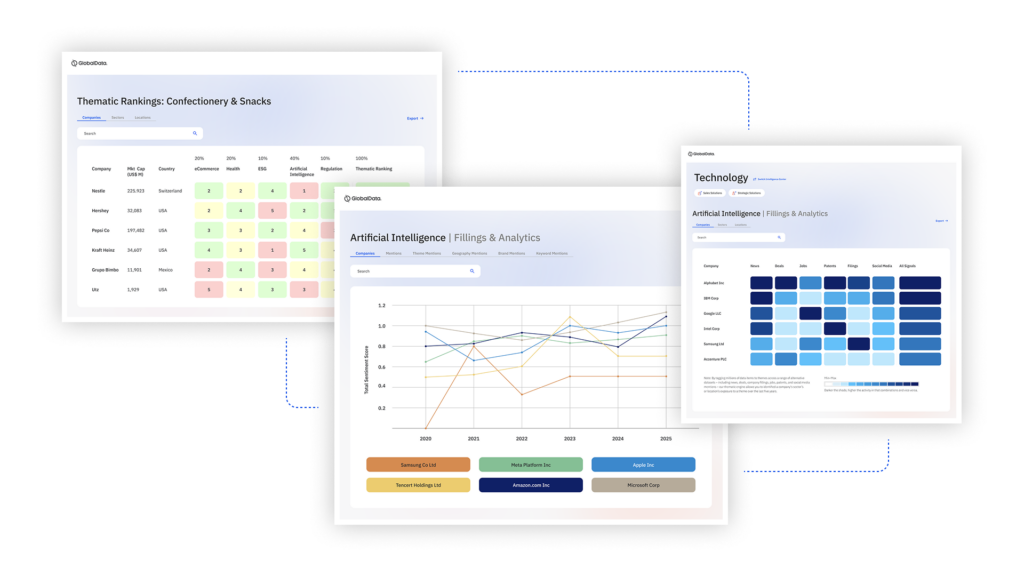
US Tariffs are shifting - will you react or anticipate?
Don’t let policy changes catch you off guard. Stay proactive with real-time data and expert analysis.
By GlobalDataIt may have been until fatal accidents involving autonomous vehicles grabbed the headlines worldwide; now there is more competition to deliver the highest level of validation before a vehicle turns a wheel on the highway. This re-alignment of priorities is good news for all parties because it will accelerate improvements in CAV safety. Autonomous developers already use proving grounds to test in mock urban environments with soft foam-targets to ensure a vehicle is safe but simulation can greatly accelerate the rate at which experience is accumulated. Early adopters of the technology are already racking up more than two million miles of testing per month.
So is a digital proving ground primarily to improve road safety?
Safety is a major driver but there is also the issue of comfort for CAV occupants. Current efforts are largely directed towards the avoidance of other road users, but for CAVs to achieve widespread consumer acceptance, they must provide a comfortable and reassuring experience for the vehicle occupants. An experienced human driver doesn’t drive in a straight line but plots a path around potholes and broken surfaces, and reacts progressively to changes in distant traffic lights, or anticipates the actions of merging traffic. Consumer acceptance must be built into the learning process, for example by using cost functions and reward functions for ride comfort and head toss KPIs. Most proving grounds have ride comfort areas that are suitable for both training and testing the AI driver models. Take robotaxis as an example. Huge amounts of venture capital are funding the race to get the first robotaxis on to the streets, with potentially massive rewards for the winner of this race. Most of us will be tempted to try a robotaxi at least once, but their business models require us to be wholly convinced by the experience. As consumers we will only take a second ride if we feel both safe and comfortable. Using a driving simulator, with a digital twin, allows you to involve human test drivers in the development process as early as possible, ensuring that the AI models you are building will achieve consumer acceptance.
How do you achieve a convincing simulation?
In order to be effective as a development tool, the simulation must correlate exactly with the real world, including the characteristics of the road surface. Clearly this is essential for the simulation of ride comfort. We utilise phase-based laser scanning survey data to create our TerrainServer surface models with an accuracy of around 1mm in Z (height) and in X and Y (position). By capturing detailed surface information that is missed by point-based sampling methods, TerrainServer allows very high correlation with the actual road surfaces used during ‘real world’ testing. Unlike other virtual models, we use exceptionally high quality, realistic rendering, up to HDR-32, in real time which is essential for the training, testing and validation of deep-learning based ADAS and autonomous systems. Our system is also unique in avoiding the patterns and video artefacts that arise in synthetic simulation tools, which would otherwise impair deep learning training performance by over-fitting the model.
Is it easier to convince the CAV systems that what is in front of them is real, compared to us humans?
The key to success is a high level of accuracy when replicating the real world in simulation, which enables the various sensors used on CAVs to react naturally, making the test results completely representative.
Not really – simulation has to test the reactions of the sensor systems to various inputs under the most challenging conditions. The key to success is a high level of accuracy when replicating the real world in simulation, which enables the various sensors used on CAVs to react naturally, making the test results completely representative. Lighting, for example, is modelled accurately for latitude and longitude, day of the year, time of day, atmospheric and weather conditions. This includes circumstances such as the transition between poorly-lit and well-lit roads, the effect of the sun low in the sky or the approaching headlights of oncoming traffic, all of which can be particularly challenging for ADAS and autonomous vehicle sensors.
But doesn’t simulation take important random human input out of the equation?
It doesn’t have to. Human drivers, cyclists or pedestrians, with their variable and unpredictable behaviour, can be an active part of the simulation. The autonomous industry now recognises the safety benefits of testing in simulation, however that does not mean you must stop testing with humans. For example, our driving simulation allow up to fifty human drivers to join each experiment, by using driving simulators or workstations with head-mounted VR systems. This means you can actually push your AI really hard, in the most complex situations, surrounded by other human road users, without any risk to life. It may seem counter-intuitive but simulation actually enables humans to get involved earlier in the development cycle because it allows them to interact with CAVs and help them to learn in a completely safe, virtual environment where nobody can be killed or injured.