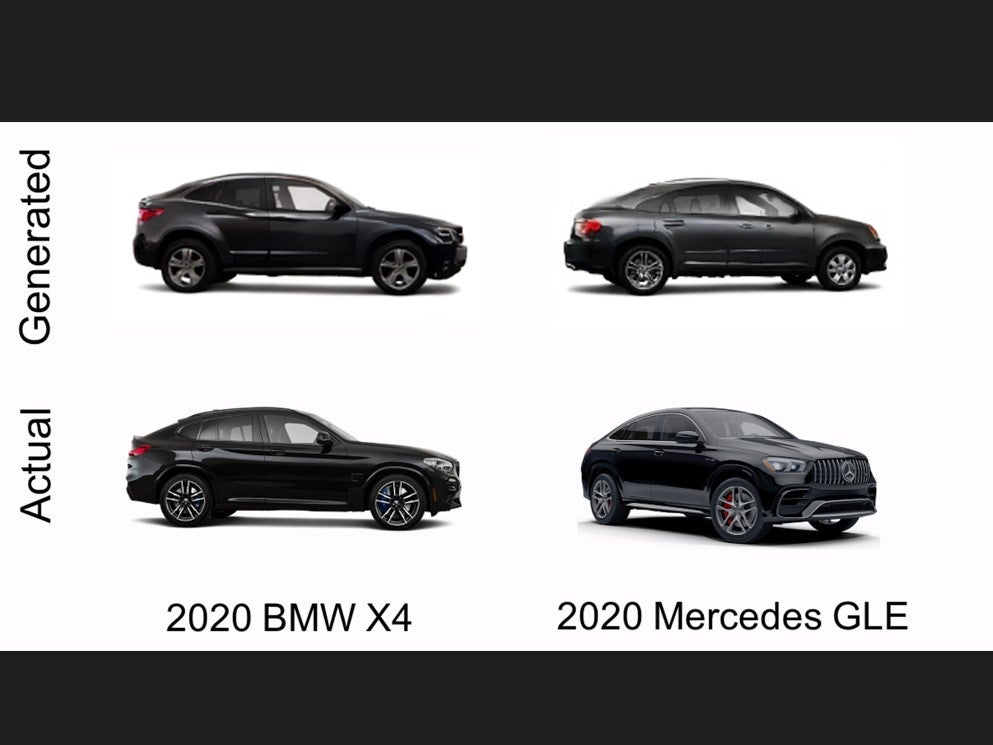
Product aesthetics have been linked to 60% of purchasing decisions in the automotive industry. With this in mind, creating an aesthetically pleasing vehicle is imperative to achieving high sales.
At present, the design process for a new vehicle is a lengthy, cost claiming process with many designs not making it through the initial stages, sending designers back to the drawing board.
A paper recently published by professors from Yale School of Management and MIT Sloan demonstrates that the use of machine learning can assist designers in predicting the appeal of new aesthetic designs, as well as to generate designs which are aesthetically pleasing.
We spoke to Alex Burnap, Yale School of Management professor, to learn more.
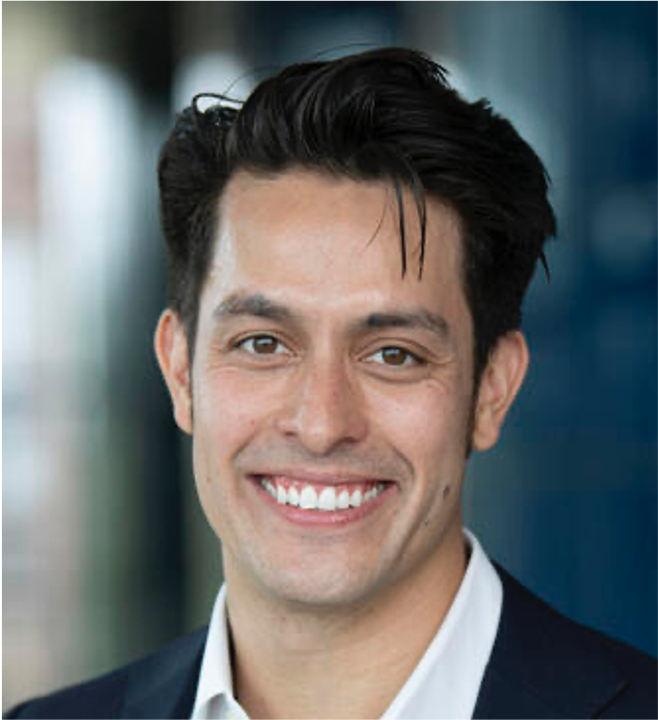
Just Auto (JA): Could you tell me a little bit about your profession?
Alex Burnap (AB): I’m currently a professor at Yale where my work focuses on improving product design and product management using artificial intelligence. Throughout my life though, automobiles have been a kind of constant narrative. I got into cars at a young age and got to be a mechanic for a bit. When I went off and did my degrees, I originally thought I was going to work on car engines. However, I got into optimisation and machine learning – but I kept in touch with cars. This was all in engineering and computer science, but I later transitioned to a business school because I wanted to have real impact on companies.
I worked for General Motors for a bit, and we were working on the key problems surrounding new product design. I’d say I have been working on helping designers design better products, in this case cars, using machine learning tools for about a decade.
My view on machine learning and AI in product design is that, especially in those early stages of the design, you can’t automate very much. Design is still a fundamentally creative process, and to have impact, you really have to help the people that are already really good at what they’re doing.
Where did the idea for this project come from?
I’ve been working for many years now on the area of product aesthetics. Even though aesthetics makes up to 60% of the purchase decision, it’s historically been really hard to use quantitative methods to help out like we do in many other parts of the design process.
For example, in engineering we have very good and widely adopted methods such as computer aided design (CAD), computational fluid dynamics, and finite element analysis, and different types of quantitative models and simulations to predict what isn’t and what will be crashworthy for example. With aesthetics it’s a lot tougher.
The key challenge with aesthetics is it’s a very high dimensional problem: If you think of all the ways you can describe an Audi or any other car, you might start off with proportions like lengths, widths, and heights, but then you might zoom in to the surfaces and the details. There would be hundreds or thousands of things one could say about the different curves and body lines—these would be the dimensions or variables that go into a quantitative method.
The key challenge with aesthetics is it’s a very high dimensional problem.
Add on top of that the fact that there is the complex and holistic relationship amongst these variables, the Gestalt if you will, in which the whole is greater than the parts, and you can quickly see how dimensionality is a major challenge.
That poses a really big problem for quantitative models, particularly market segmentation and new product design models, because you’re likely dealing with millions of relationships between variables to tease out what customers within the overall market will actually want. Traditional quantitative models excel in lower-dimensional problems, say, predicting how customers trade off horsepower and fuel efficiency, and how much they are willing to pay. With aesthetics, because you have so many more dimensions, so many more variables to account for, it’s too high dimensional for the typical marketing and product design models and that’s where the machine learning side comes in.
This is in many ways a natural progression. I wouldn’t say the use of computational methods to improve how we design new products is particularly new; for example, CAD tool from 1980s were a major improvement what was then the traditional design process. However, as we have shown in our work, recent advances in generative AI have opened new opportunities for improvement. This is particularly true for the early stages of design—the aesthetic design process—which has been an open problem because of the high-dimensionality issue.
How can this technology assist the automotive market when it comes to design?
One way is to think of the new product design process as a series of iterative refinement, from initial target market definition, to design generation and testing, and to engineering and manufacturing to launch the final product. At the earliest stages the product design is very loose in its form. There will be hundreds or thousands of possible design concepts, initially sketches from designers, which get whittled down. A lot of it is thrown out and there’s a lot of validation along the way.
Aesthetic design is not a linear process at all, it’s heavily iterative between the generation and the testing of possible product designs. Generation is driven by the designers themselves, those sketching it out, as well as design and brand managers. Testing is often the purview of marketing and product research. There you’re bringing customers in and taking all these possible design concepts and trying to test out which ones are going to work. This relies on pretty expensive focus groups, in the industry called ‘Theme Clinics’, where the brand brings in 100’s of very well-targeted customers for a week and shows them different designs to hopefully predict what the overall market is going to want. Our work focuses on these two early stages—design generation and design testing.
Aesthetic design is not a linear process at all, it’s heavily iterative between the generation and the testing of possible product designs.
In both the design generation and the design testing stages, a key emphasis of our work is that you need to really view these as tools to augment, not automate. Robots aren’t taking the jobs. The machine learning method we developed helps the humans involved in generation and testing.
This was a key insight. On the design generation side, we initially thought the goal was a tool that makes “realistic-looking” possible designs—this is the focus of research in generative AI, but it’s not what designers wanted and would truly benefit from. This lead to the augmentation idea. Designers wanted fine grain generation control; they wanted it as a tool to allow them to be more creative.
We made a lot of modelling decisions that actually reduced the realism of generated designs, but with the trade-off of making this AI tool much more controllable while still being “realistic enough”. We’re giving the designers tuning knobs so they can ask questions they want answered. And it puts these tools in the hands of designers so they can control them in a private way independent of the market research teams, so they don’t feel like they’re being graded. More generally and perhaps obvious in retrospect, getting useful applications of AI into companies is more than fancy AI tools, but deeply understanding what problems the company faces and how to fit into existing workflows.
What benefits does this offer over the traditional method of design?
On the design testing side, when market researchers run focus groups, they are expensive and they’re going to run for a long time. The augmentation with machine learning says: Hey, let’s do what you’re already doing but rather than taking these hundreds of different possible design paths, we can “pre-test” a whole bunch of them so you can focus on the best ones. The benefit is that this results in major time and cost savings, and increases the throughput of number of designs considered to find potentially better ones than the traditional approach.
On the design generation side, the benefit is increased exploration of the space of possible designs. Designers can creatively explore product forms that they might not have otherwise, some of which may end up being major market successes. We developed the AI method to fit this benefit. One key area was the aforementioned controllability versus realism trade off; this is what designers wanted and would actually use. Another was to allow the model to be separable and usable independently. So, the benefit is it’s practical for use in the sense that design is very iterative and some design teams might be working on one version of the design, and others might be working on others.
In short, designers are churning out more creative designs, testing groups are testing more designs and knocking out the bad ones to focus on the best ones, and the whole process gets improved.
What do you see the future holding for automotive design?
I think there are several major changes that will happen, but not necessarily overnight. Artificial intelligence and machine learning may be advancing at a rapid pace, but practical uptake to solve real business problems is a perhaps a harder problem. It requires domain-specific knowledge at the intersection of both AI and the company’s domain, as well as overcoming the inertia of existing company workflows. This takes time.
While recent advances in AI are perhaps not widely adopted yet, I know many companies and their leaders are interesting in how AI can improve business outcomes. I think it’s safe to bet that adoption into companies will naturally happen faster once the value of AI tools prove themselves more broadly across product categories, including the automotive industry. At some point the competitive market, and specifically your competitors’ AI-improved processes leading to launching successful products, will make it impossible to ignore.
Focusing on the aesthetic design problem we’ve tackled, I think you’ll see adoption of AI methods like the one we developed be heavily dependent on the company and the people within them. On the technical side, early adopters are likely the larger companies that can afford R&D divisions. You need people that understand both the technical side of machine learning as well as deep knowledge of actual company problems and use cases. On the people side, early adopters are those that are positioned to be open to new workflows. In my experience this is particularly the younger designers, as well as design managers that are very open minded, and I think many of them are.
Lastly, an important trend with the automotive industry itself is the amount of data on both product usage and consumer behaviour that is being generated by today’s cars. This is the sort of data that is the fuel for AI engines. It may be interesting to recognize the car, traditionally a hardware product, is currently transitioning to more of a hybrid hardware-software product. Look at Tesla‘s for instance: They’re sending over-the-air updates like you would with updating your smart phone. These aren’t just firmware security updates, but change the fundamental nature of cars’ driving dynamics and consequently how we as consumers interact with the product. I see automobiles becoming more hybrid in that way, as data platforms themselves. This has significant implications on the company side for improving new product design and market success, but perhaps moreso, the role that cars ultimately play in our lives.
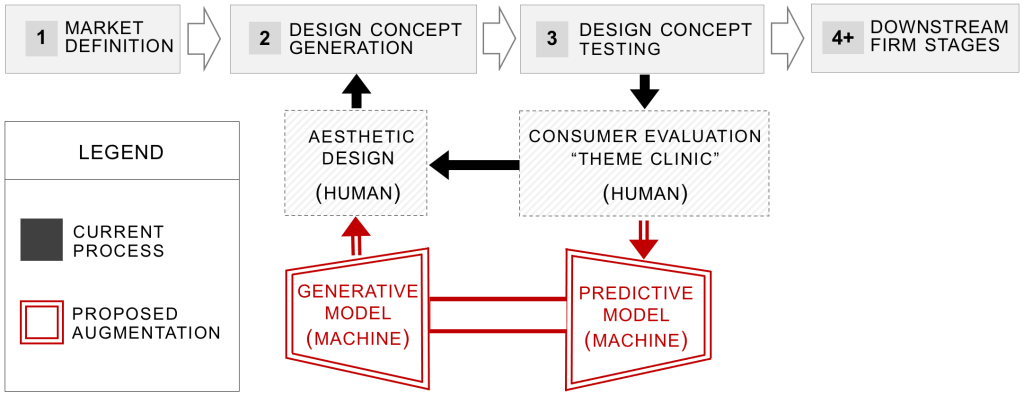