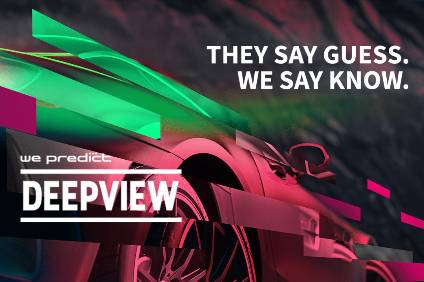
Formed in 2009, 'We Predict' is a company using machine learning and predictive methodology to leverage forecasts from big databases. The US-based company is targeting a number of industries for its services, including automotive. In the UK, a team of data scientists, software coders and mathematicians works on a product utilising automotive warranty data (NA) that it claims can predict automotive parts failures by make and model. Dave Leggett visited their Swansea offices to find out more.
The UK- and Ann Arbor-based firm says its predictive methodology can accurately predict component repairs and replacements when vehicles have been in service for 90 days, one year, two years, three years or longer.
"Automakers for decades have had varying degrees of information to understand the recent and historical warranty performance on their own products," said Renee Stephens, vice president of automotive operations at We Predict. "Incredibly, there was little, if any, way for them to understand how they fared competitively on repairs."
Stephens, who previously managed automotive studies for market research firm JD Power, notes that customer surveys have their limitations as they shed insight only on what has happened in the past – not predict what will happen in the future – and rely on an owner's memory of what was fixed on their car.
"If they can see today that their parts are expected to be replaced at four times the rate of the competition, and two-to-three years ahead of those customers experiencing the repair, the automaker can take action now to mitigate the problem," Stephens said. "Having this forward-looking and competitive perspective is especially important as companies are launching new technologies. They don't have the luxury of waiting years for trends to mature."
The We Predict study is called 'Deepview' and it utilises millions of records for warranty and customer pay work to take the guess-work out of predicting automotive warranty repairs. Furthermore, the study allows automakers and suppliers and others to assess part repair and replacement rates, not only for their vehicles or components, but across the industry.
How well do you really know your competitors?
Access the most comprehensive Company Profiles on the market, powered by GlobalData. Save hours of research. Gain competitive edge.
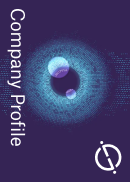
Thank you!
Your download email will arrive shortly
Not ready to buy yet? Download a free sample
We are confident about the unique quality of our Company Profiles. However, we want you to make the most beneficial decision for your business, so we offer a free sample that you can download by submitting the below form
By GlobalDataThe study features predictive component repairs for all major brands (45 total current and more historical brands) and all segments in the US, and more than 500 models, including many electric and hybrid variants, and spans many model years. Much of the database development work, though, happens in the UK – in Swansea, on the coast of South Wales.
At the Swansea office, Dr Stephen Norris acts as technical lead, with Juliet Quantrill liaising with business customers. Swansea is a coastal town in South Wales and the home of a university that churns out the kind of young professionals that We Predict needs.
The basic product relies on a very large data set of vehicle repairs and We Predict has put considerable resource into developing that data set, sources and the relevant expertise to analyse the data. "You need the data and you need the techniques," says Norris. "The two things very much come together."
Norris sees a sweet spot for this repairs data. "When we speak to the OEMs, they want to know how they compare with their competitors. And of course, it's the same for the suppliers. They want to know where they rank."
It's a benchmarking tool and We Predict has been doing this type of analysis with data for a long time, Norris says. They take a data set involving warranty claims, sales data and vehicle population and usage information and turn that into "detailed predictive analytics".
"The predictions can be at the car-line level, brand level, vehicle sub-system level, or the component level. We can forecast for every single combination or permutation in the data the behaviour of a vehicle or component when it reaches the end of its warranty."
"The predictions can be at the car-line level, brand level, vehicle sub-system level, or the component level. We can forecast for every single combination or permutation in the data the behaviour of a vehicle or component when it reaches the end of its warranty," he says.
There must be a lot of work to standardise or normalise data that comes in from different sources? "Yes, there is a lot of work to make sure that the data is coherent but we have a lot of experience with the data. At one time or another we've worked with all of the major OEMs. We work with lots of suppliers. We understand the warranty space and data really well. We're in a unique position to take all that data and makes sense of it, normalise it, so that it is all comparable. If it's not comparable, then people will dispute the numbers straight away. It has to make sense. And in this industry people use different terms for the same components, so you have to know the data quite intimately. My background is automotive, so I'm well aware of things like that."
The warranty data is US only. "We could expand to other countries if more data became available," he says. "But the good thing about the US data is that it's a very large market and we have a large volume of data; it is a highly representative data set due to its size."
There is relevance beyond North America though. "It links back to Europe because many of the vehicle lines and components are made in Europe as well. So as well as US companies being interested, so are European ones who have their components or vehicles in that data set."
It's all very intriguing, but I can't help wondering why other well known US data providers – Polk springs to mind – are not in this space.
Juliet Quantrill, who works in a business development role for We Predict, homes in on the key factor: Yes, it's the clever coding. "It's the specific algorithms that we developed, right from the early days. That's our IP and it is completely tied down. And that's also why there is so much interest in us as a company from investors. As far as we know there is no other company able to analyse to the accuracy that we can achieve."
Insurance company Munich Re is an investor and there's a natural synergy with the world of insurance. "They have helped us and reinforced our credibility. We can offer warranty risk insurance to our customers based on our numbers and forecasts. As well as providing forecasts on components that help engineers understand relative failure rates for components, we can take all that data, aggregate it to budget years and replace failure frequencies with cost frequencies you can start to predict what your [OEM] warranty accrual needs to be for the next two, three, four years. What we can then do with those numbers, with a reinsurance company like Munich Re, is offer an insurance package to a company. We'll tell them what we think their accruals need to be. If they go a certain level above that, Munich Re pays the difference."
So the insurance company's involvement acts as a kind of underwriter for the We Predict forecast risk? "Yes. Not only is it another product we can offer, but their involvement adds to our credibility in the market. They [Munich Re] obviously have to be happy with our numbers to insure that level of risk."
"As the data comes in, the models that are needed to run the forecast get built automatically."
How does the database work? I am interested to hear some process basics – while not being blinded with science, if possible. Norris helpfully obliges: "The data comes in continuously from clients (companies) – comprising warranty claims and sales data. It can be refreshed weekly, monthly (monthly cycles are common), and as the data comes in, the models that are needed to run the forecast get built automatically. That is tied together in a hierarchy that takes all the current vehicles that are out there working under warranty and we look at their performance now in detail – number and nature of claims, costs down to component level – and then we forecast each of those elements out into the future – 12 months ahead, 24, 36, 48, depending on the warranty length that people are interested in."
This forecasting methodology, Norris says, allows a fair comparison against known – and different – model cycles. "We are forecasting to the same relative point in time for each vehicle which takes account of different launch times," he maintains. "This is absolutely key because it normalises all the data, so if a Honda Accord launches in April and Ford Focus launches in October, without systematic forecasting it would be very difficult to align those two vehicles for a fair data comparison. By forecasting them both out to say three years' warranty, they are suddenly at the same relative starting point. You get a proper idea of which one has the best or worst forecasted reliability."
Intriguing stuff. As more claims data comes in, the forecasts become better and they can track the variance of actual data to forecast. "We can show people how the forecast changes. As more data comes in, we can see that the forecast becomes more stable," Norris points out. "As the model year progresses we can get a really good feel for the data and more confidence in the forecast. The key is understanding the data. We only forecast when the claims come in. It's about maturity of the vehicle population on that particular model line and what we think the claims will turn into as vehicles age through the lifetime of the model."
The models are driven by historical claims data on the model concerned and similar models from the same OEM that have been through warranty. "A lot of people don't look at the data like this and they are missing the really rich seams of information that are in it."
Stable data that follows predicted patterns is reassuring, but Norris says erratic data can also be informative. "A sudden spike shows that something is happening, so the challenge then is to understand what may have changed. The key thing is to have the data, understand it and its limitations. The beauty of what we have is that there is a vast quantity of data and we can see how the forecasts track as more actual data comes in. There is a normal warranty pattern that informs much of our modelling. If the current behaviour is in line, then the warranty pattern for vehicles out there now will be similar. If something is changing a lot, deviating significantly from our model or inexplicably spiking, that is also good to know. It tells us something and that can be a very valuable conversation to have with a customer."
It sounds like a refreshing attitude; data is king but this isn't just about data points in the future. Understanding and interpretation is key in a dynamic process that is constantly informed by the latest data. A look at the company website reveals that the data science at work is being applied to other sectors, too, including healthcare and sports. It could be a company to watch.